Unlocking the Power of Image Annotation: The Best Practices to Label Images for Object Detection
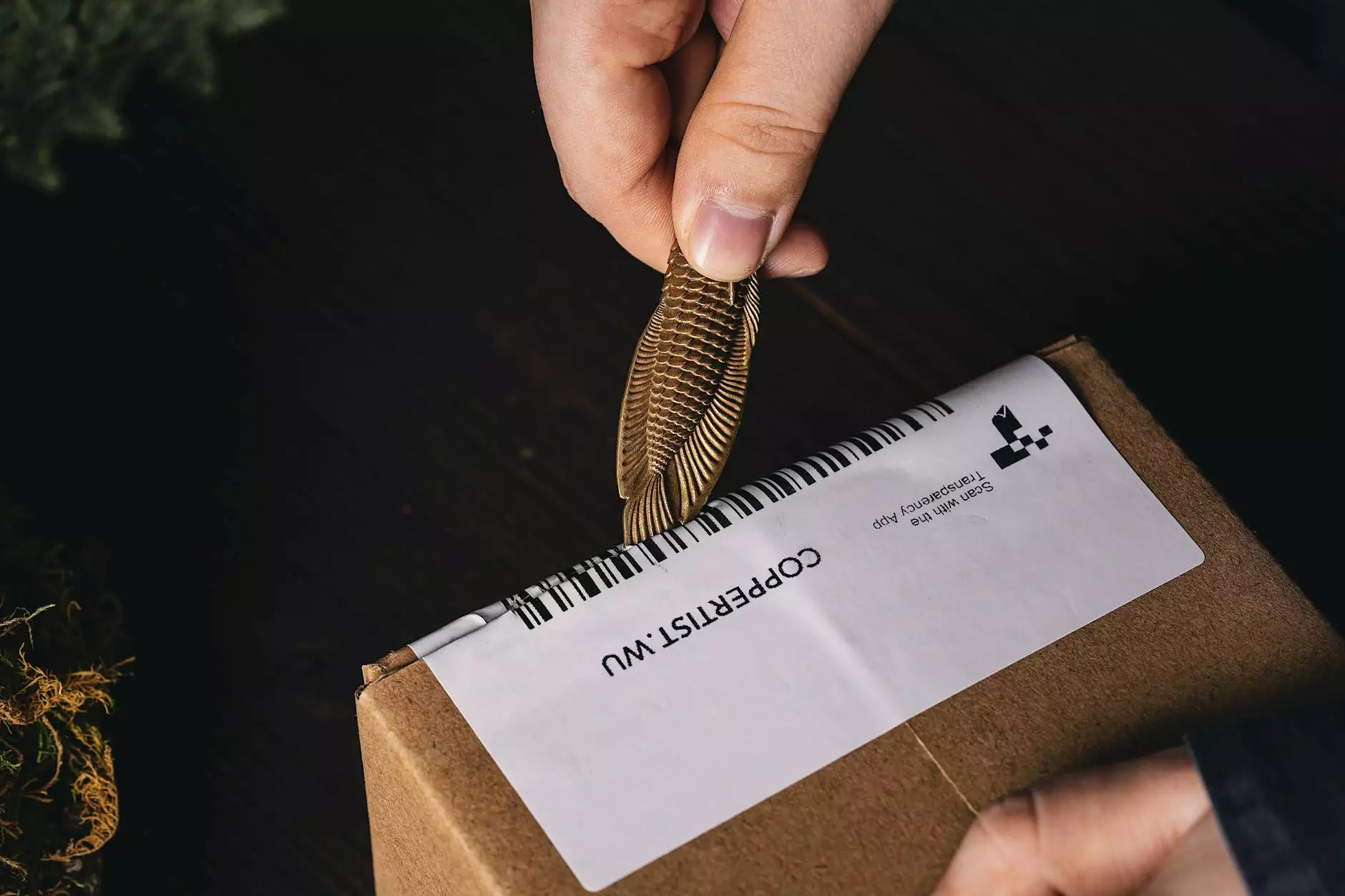
In the realm of artificial intelligence and machine learning, data annotation plays a critical role, particularly when it comes to labeling images for object detection. Object detection is a technology that enables machines to identify and classify various objects within an image, making it essential in various applications from autonomous vehicles to security systems. This article will provide you with an in-depth look at the processes involved in labeling images, the tools available, and how Keylabs.ai can facilitate this pivotal task.
Understanding Object Detection
Before diving into labeling techniques, it’s crucial to understand what object detection is and its significance in today's technological landscape. Object detection aims to not only identify objects within images but also to locate them effectively. This process is employed in various sectors, including:
- Automotive: Powering self-driving technology.
- Healthcare: Assisting in diagnostics through imaging.
- Security: Enhancing surveillance systems.
- E-commerce: Improving product discovery.
The Importance of Data Annotation
Data annotation acts as the essential foundation for training machine learning models. Without accurately labeled data, these models can produce unreliable and inaccurate outputs. Here are some reasons why quality data annotation matters:
- Accuracy: Detailed labeling enhances model accuracy.
- Efficiency: Provides a more streamlined training process.
- Scalability: Facilitates easy model scaling as new data comes in.
Labeling Techniques for Object Detection
When it comes to labeling images for object detection, various techniques can be employed. Let's explore these methods in detail.
1. Bounding Boxes
Bounding boxes are the most common method for labeling images. A box is drawn around the object of interest, identifying its location within the frame. This technique involves:
- Selecting an image.
- Defining the box around the object.
- Assigning a label to the entire box.
Bounding box annotations are widely used due to their simplicity and effectiveness in training models.
2. Semantic Segmentation
This technique involves labeling each pixel of an image. It is more detailed than bounding box annotations and is particularly useful for tasks requiring a high level of precision, such as medical imaging and video segmentation.
3. Instance Segmentation
Instance segmentation combines object detection and semantic segmentation. Different objects of the same class can be distinguished at the pixel level. This technique is essential when multiple instances of the same category appear in an image.
4. Keypoint Annotation
This method is mainly used in applications requiring the identification of specific points on an object, such as facial recognition or pose estimation. For example, labeling facial landmarks assists in tracking expressions and movements.
Choosing the Right Tools for Data Annotation
Selecting the appropriate tools is critical for efficient and effective labeling of data. Here’s how Keylabs.ai stands out:
The Keylabs.ai Advantage
At Keylabs.ai, we provide a comprehensive Data Annotation Platform that is user-friendly, scalable, and designed to meet diverse business needs. Here are some of the highlights:
- Advanced Annotation Tools: Our platform supports various annotation types including bounding boxes, polygons, and semantic segmentation.
- Customizable Workflows: Tailor your annotation workflow to meet the specific needs of your project.
- Collaboration Features: Enable collaborative work among your team members for more efficient data processing.
- Quality Control: Several quality checks ensure the precision of annotations, leading to better model training.
Best Practices for Labeling Images for Object Detection
To ensure the quality and usability of your labeled data, follow these best practices:
1. Consistency is Key
Keeping a consistent labeling format and guidelines across your team is vital. This will prevent discrepancies and provide a uniform dataset for model training.
2. Use High-Quality Images
The quality of your dataset directly impacts model performance. Use high-resolution images to enable more precise annotations.
3. Create Clear Labeling Guidelines
Establish comprehensive guidelines that define how different categories should be labeled. This will help new annotators and maintain consistency across the dataset.
4. Review and Adjust
Regularly review the labeled data and adjust annotations as needed. Continuous quality assurance can significantly improve the performance of your models.
The Future of Data Annotation in Object Detection
As technology continues to evolve, so will the methods and tools for labeling images for object detection. The rise of automation and machine learning techniques is set to change the landscape of data annotation. Here are some trends to watch:
- Automation: Increasingly, tools will incorporate AI to automate the labeling process, reducing time and cost.
- Real-time Annotation: Technologies enabling real-time data annotation will become more prevalent in practical applications.
- Cloud-based Platforms: With the shift to cloud computing, data annotation tools are becoming more accessible and scalable.
Conclusion
Labeling images for object detection is an indispensable step in the world of AI and machine learning. By utilizing effective techniques, choosing the right tools, and adhering to best practices, businesses can significantly enhance their data annotation processes. Keylabs.ai offers the sophisticated solutions necessary to meet your data annotation needs, ensuring that your models are trained on high-quality, accurately labeled data. Embrace the power of meticulous image annotation today and watch your AI capabilities soar!
label images for object detection